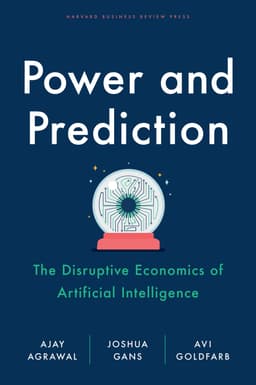
Power And Prediction Book Summary
The Disruptive Economics of Artificial Intelligence
Book by Ajay Agrawal, Joshua Gans, Avi Goldfarb
Feeling Lazy?Read 1 Minute Summary
Summary
"Power and Prediction" argues that the true potential of AI lies not in automating individual tasks, but in enabling the redesign of entire systems and decision-making processes, which will lead to significant shifts in economic and political power as AI evolves from a tool for prediction into a catalyst for transformation.
Sign in to rate
The Parable Of Three Entrepreneurs
The authors use the historical example of the slow adoption of electricity to illustrate the challenges of deploying a new general purpose technology like AI. They describe three types of entrepreneurs that tried to exploit electricity in different ways in the late 19th/early 20th century:
- Point solution entrepreneurs who simply replaced steam power with electric power with minimal factory redesign. This provided limited benefits.
- Application solution entrepreneurs who redesigned individual machines and tools around electric motors. This enabled some new capabilities but still limited benefits without factory redesign.
- System solution entrepreneurs who completely redesigned factories to fully exploit the unique advantages of electric power. This is what ultimately transformed manufacturing and the economy, but it took decades.
Section: 1, Chapter: 1
AI Adoption Faces The Same Challenges As Past General Purpose Technologies
The authors argue that AI is a general purpose technology (GPT) like electricity and the steam engine that has the potential to transform the economy over time. However, as with past GPTs, there is a significant delay between the initial invention and demonstration of the technology and its widespread adoption and impact on productivity. The authors refer to this delay as "The Between Times." During this period, point solutions and application solutions emerge, but the big productivity gains come only later with the development of system solutions that more fully exploit the technology's potential.
Section: 1, Chapter: 2
AI Is Fundamentally A Prediction Technology
The authors argue that the essence of recent advances in AI is that they represent a dramatic improvement in prediction - the ability to take information you have and generate information you don't have. Prediction is a key input into decision making. As prediction becomes cheaper, we will use more of it and the value of other inputs to decision making like human prediction will fall while the value of complements like data and judgment will rise. Judgment is determining the relative payoff or reward to different actions - it is a statement of what we want, while prediction tells us the likelihood of different outcomes.
Section: 1, Chapter: 3
"AIs Are Idiot Savants, Not General Intelligence"
"Predicting on the support of your data is not as simple as collecting data from a wider variety of settings to ensure you aren't extrapolating too much or avoiding predicting too far into the future. Sometimes the data you need doesn't exist. This underlies the refrain repeated in every statistics course worldwide: correlation is not necessarily causation."
Section: 1, Chapter: 3
Causal Inference Is Critical For Effective AI Deployment
When deploying AI systems, it's critical to ensure the predictions are valid for the decisions you want to make. Some key considerations:
- Distinguish between correlation and causation. AI predictions based on historical data may identify correlations that don't reflect causal relationships.
- Collect data that covers the full range of relevant situations. Predicting outside the "support of your data" is risky.
- Where possible, use randomized experiments to collect data that reliably measures causal impact. Leading tech companies now employ many economists and statisticians focused on causal inference.
Section: 1, Chapter: 3
2. Rules
Decisions Incur Cognitive Costs, While Rules Enable Reliability
The authors explain the difference between decisions and rules:
- Decisions allow you to take into account situational information but incur cognitive costs to gather information and deliberate
- Rules avoid cognitive costs but result in the same action regardless of the situation The key factors that determine if the cognitive cost of a decision is worthwhile are:
- The consequences of the decision - more consequential decisions are worth deciding
- The cost of information - if information is cheap, decisions become more attractive
Section: 2, Chapter: 4
AI Enables Turning Rules Into Decisions
As a leader, you should examine the existing rules and standard operating procedures in your organization and evaluate if they can be turned into decisions enhanced by AI predictions. Look for situations where:
- The rule leads to costly errors in some situations that could be mitigated by deciding differently
- AI can provide cheap, high-quality predictions to enable better situational decisions
- The cost savings or performance gains from better decisions justify the cognitive cost and reduced reliability of turning the rule into an AI-informed decision
Section: 2, Chapter: 4
Uncertainty Can Be Hidden By Rules And Expensive Accommodations
The authors use the example of modern airport design to illustrate the concept of "hidden uncertainty." Frequent air travelers arrive at the airport much earlier than their flights to accommodate the uncertainty around traffic, parking, security lines, flight delays, etc. Airports like Incheon Airport in South Korea now provide extensive amenities like spas, museums, gardens, and ice skating to make the inevitably long wait times more palatable.
However, this expensive infrastructure accommodates the hidden uncertainty rather than resolving it. The authors suggest that AI prediction could reduce the uncertainty and enable a new, more efficient equilibrium.
Section: 2, Chapter: 5
AI Navigation Apps Could Disrupt The Economics Of Airport Retail
Airport operators should be wary of the disruptive potential of AI-powered navigation apps like Waze and Google Maps. Key considerations:
- These apps can provide increasingly accurate, personalized predictions of travel time to the airport, reducing the need for passengers to budget large uncertainty buffers
- As passengers become more confident in "just in time" airport arrival, demand for in-terminal retail and dining may fall significantly
- Airport operators should explore ways to actively partner with navigation apps to shape behavior and preserve retail revenues, rather than being passive victims of disruption
Section: 2, Chapter: 5
Standard Operating Procedures Provide Reliability But Stifle AI-Enhanced Decisions
The authors present a framework for understanding the role of rules and standard operating procedures (SOPs) in organizations:
- SOPs reduce individual cognitive load by providing pre-specified actions
- SOPs enable reliability and predictability across an organization by ensuring different people/groups take consistent, coordinated actions without extensive communication
- SOPs "glue" different parts of an organization together in an interdependent system resistant to change
- Replacing SOPs with AI-enhanced decisions reduces reliability and predictability, unsticking the organizational glue
- Transforming rules into AI-enhanced decisions often requires systemic change to re-establish coordination in new ways
Section: 2, Chapter: 6
SOPs Are The Manifestation Of Rules And The Need To Follow Them
"Like SOPs, checklists are the manifestation of rules and the need to follow them. They are there to ensure reliability and reduce error. The alternative is that people make decisions based on their own observations. While switching from a rule to a decision may improve the quality of that particular action, it may also create problems and uncertainty for other people."
Section: 2, Chapter: 6
AI Can Decouple Education From The Factory Model Of Schooling
The authors discuss how AI could enable a transformation of the education system from the current "factory model" where students progress based on age to a personalized model where each student receives customized instruction based on their individual learning needs and pace. Key points:
- In the factory model, the curriculum is tied to the student's age and grade rather than their individual progress, and teachers deliver one-size-fits-all instruction
- AI-powered adaptive learning systems can predict the optimal next lesson for each student based on their performance, enabling them to progress at their own pace
- Realizing this vision requires not just better AI but a redesign of the education system, including changes to student grouping, pedagogy, teacher training, facilities, etc.
Section: 2, Chapter: 6
3. Systems
Covid-19 Illustrates The High Costs Of Managing Uncertainty With Crude Rules
The authors use the example of blanket social distancing rules during the Covid-19 pandemic to illustrate the economic costs of managing uncertainty with rules rather than predictions.
- Social distancing was a crude but effective rule for limiting virus spread in the absence of information on who was infectious
- However, it came at an enormous economic and social cost, disrupting work, education, and social connectivity for everyone rather than just the infectious
- Better predictive tools for identifying infectious individuals, like rapid testing, could have enabled more normal life to continue safely for the majority. Realizing this would have required an "oiled" system able to flexibly adapt based on new information, rather than a "glued" system locked into rigid rules
Section: 3, Chapter: 7
Beware The "AI Bullwhip" When Optimizing Interdependent Systems
When adopting AI to optimize different parts of an interdependent system, beware of the "AI Bullwhip effect" when the output of one AI-optimized component becomes the input to another in an unanticipated way. For example:
- A restaurant adopts an AI system to predict food orders and optimize its inventory, reducing waste
- This makes the restaurant's orders to suppliers less predictable, forcing suppliers to carry more inventory
- The added volatility ripples through the supply chain, forcing each tier to adopt its own
AI optimization Leaders should carefully map out interdependencies in their systems and plan for the impact of AI optimization on upstream and downstream components.
Section: 3, Chapter: 8
Task-Level Thinking Misses AI's Potential To Drive Organizational Redesign
The authors argue that the predominant "task-level" paradigm for thinking about AI adoption and impact is misguided and limiting. The key points:
- Most leaders and experts focus on identifying specific tasks that AI could perform better than humans and calculating the labor substitution effects
- However, the greatest value from AI comes not from piecemeal task substitution but from reimagining entire systems and processes around AI capabilities
- Focusing on tasks leads to small-scale point solutions, while system-level thinking enables transformative new structures and strategies
- The biggest AI successes to date, like Netflix, Amazon, and YouTube, have come from system-level innovation rather than task substitution
Leaders should adopt a "system mindset" and focus on how AI predictions could enable fundamentally new approaches to delivering value, even if those approaches are inferior on traditional metrics.
Section: 3, Chapter: 8
AI Is Revolutionizing The Innovation Process Itself
The authors argue that AI's most profound impact may be on the process of innovation and invention itself. Key points:
- AI enables faster and cheaper hypothesis generation and testing, accelerating the innovation cycle
- AI-powered tools like AlphaFold are dramatically reducing the time and cost of key innovation steps like protein structure prediction
- Just as previous research tools like microscopes enabled the discovery of the germ theory of disease, AI is a "new method of invention" that will have cascading effects on multiple domains
Section: 3, Chapter: 9
AI Enables Innovations To Be Tested Via Simulation
The authors describe how AI is enabling innovators to test ideas via simulation rather than costly real-world experiments.
- Pharmaceutical companies can use AI to predict the outcomes of clinical trials, enabling them to prioritize the most promising drug candidates
- Aerospace companies can use AI-powered simulations to test new aircraft designs without building physical prototypes
- E-commerce companies can use AI to simulate the impact of website changes on customer behavior before deploying them live
By making experimentation faster and cheaper, AI simulations accelerate innovation and reduce risk. However, moving too quickly from simulation to the real world can be dangerous, as the fatal accidents involving Boeing's 737 Max and Tesla's self-driving systems illustrate.
Section: 3, Chapter: 9
4. Power
The AI Innovator's Dilemma Favors Startups Over Incumbents
The authors argue that AI presents an "innovator's dilemma" that makes it difficult for incumbent firms to adopt the technology in a timely and effective manner. The key challenges:
- Incumbent firms are optimized for their existing systems and metrics, while AI often requires new systems and metrics to deliver value
- Adopting AI may cannibalize incumbents' existing profitable businesses, while startups have no such conflicts
- Incumbents' organizational structures and processes are "glued" together in ways that resist the changes required to fully exploit AI
AI systems often decouple prediction from judgment in ways that disrupt incumbents' existing decision-making structures and power dynamics As a result, the authors expect AI to be more rapidly adopted by startups than incumbents, leading to significant disruption as new entrants scale up AI-powered systems that incumbents struggle to match.
Section: 4, Chapter: 10
Machines Don't Actually Make Decisions, Humans Do
When deploying AI systems, it's critical to remember that machines don't actually make decisions, humans do.
- AI systems automate the execution of decisions, but the judgment embedded in those systems always comes from humans
- The humans who specify the objectives, metrics, and reward functions for an AI system are the real decision-makers, not the system itself
- Responsible AI deployment requires carefully specifying the judgment an AI system should execute, anticipating edge cases, and monitoring for unexpected behaviors
Leaders should ensure clear human accountability for the decisions executed by AI systems, rather than allowing those systems to be treated as autonomous agents.
Section: 4, Chapter: 11
AI Increases The Returns To Scale And Scope
AI is increasing the returns to scale and scope in many industries, creating opportunities for companies to build significant competitive advantages. Some key strategies:
- Invest in proprietary data assets that enable your AI systems to make better predictions than rivals
- Build feedback loops that allow your AI systems to learn and improve over time, increasing their predictive edge
- Look for opportunities to apply your AI-powered predictions in adjacent markets and product categories
- Exploit the "flywheel effect" by using AI to attract more users/customers, generating more data, leading to better predictions, in a virtuous cycle
However, be aware that these same dynamics can help rivals build insurmountable leads if they get ahead in the AI race. In industries with strong AI feedback loops, being a fast follower may not be a viable strategy.
Section: 4, Chapter: 12
5. How AI Disrupts
AI Enables The Decoupling Of Prediction From Judgment
The authors argue that one of AI's most profound effects is to decouple prediction, which is increasingly done by machines, from judgment, which remains the province of humans. Key implications:
- Prediction and judgment are the two key ingredients of decision-making; before AI, they were tightly coupled in the minds of human decision-makers
- AI allows prediction to be centralized and automated while judgment remains decentralized and human-driven
- This decoupling creates opportunities to reimagine systems and processes, but also creates challenges around aligning predictions with appropriate judgment
- As AI takes over more prediction, the key differentiator for human decision-makers will be their judgment, i.e. their ability to specify what objectives should be maximized
Section: 5, Chapter: 13
"Decoupling Creates A New Opportunity For AI Adoption"
"Once the AI provides the prediction, then the people with the best judgment can shine... Once the AI provides the prediction, new systems can arise to take advantage of better, faster, and cheaper predictions and more appropriate judgment. In Prediction Machines, we highlighted an opportunity for Amazon to change its business model so that it ships items to your door before you even order. That business model now exists. Stitch Fix does it for clothes."
Section: 5, Chapter: 13
AI Requires A Shift From Deterministic To Probabilistic Thinking
Adopting AI requires a shift from deterministic to probabilistic thinking in decision-making. Some key mindset changes:
- Embrace uncertainty and accept that all predictions are probabilistic, rather than expecting definitive answers
- Think in terms of expected value, weighing the probability of different outcomes rather than trying to eliminate all risk
- Be transparent about the confidence level of predictions and the potential for error, rather than presenting predictions as certain
- Build processes to periodically retrain models and update predictions as new data becomes available
- Develop ethical frameworks and oversight mechanisms to ensure predictions are applied with appropriate human judgment
Section: 5, Chapter: 14
AI Shifts Power To Those With The Best Judgment, Not The Best Predictions
The authors illustrate AI's impact on the allocation of power with the case of the Flint, Michigan water crisis.
- City officials initially ignored data showing high lead levels in the water supply, relying on flawed testing and flawed judgment
- Outside researchers used AI to predict which homes were likely to have lead pipes and successfully pressured officials to target remediation efforts based on their predictions
- The researchers' models were technically superior to the city's testing methods, but the key factor was that the researchers had better judgment about how to act on the predictions
- Ultimately, power over the response shifted from city officials to a court-appointed monitor, who had the authority to override officials' flawed judgment and act on the researchers' predictions The case illustrates how AI can shift decision-making power to those with superior judgment, even if they don't have the best predictions or the formal authority.
Section: 5, Chapter: 15
6. Envisaging New Systems
The AI Canvas For Redesigning Decisions And Systems
The authors present an "AI Canvas" framework for mapping how AI predictions could enable businesses and organizations to redesign their decision-making systems. The key steps:
- Define the core objective or "north star" of your organization
- Identify the key decisions required to achieve that objective, assuming the availability of perfect predictions
- For each decision, specify the prediction required as an input and the judgment required to act on that prediction
- Analyze how the decision-making roles and processes would need to change to incorporate AI predictions
- Redesign the overall system to maximize the value of AI predictions while preserving necessary human judgment
The authors apply the framework to an extended case study of the insurance industry, showing how AI could transform insurers' decision-making from underwriting and claims processing to customer acquisition and retention.
Section: 6, Chapter: 17
AI Bias Often Reflects Flaws In The Underlying System
The authors argue that AI bias is often a reflection of flaws in the underlying system rather than a problem with the AI algorithm itself. Key points:
- AI predictions are only as good as the data used to train the algorithm; if that data reflects historical biases or discrimination, the predictions will too
- Fixing AI bias requires not just tweaking the algorithm but also addressing the root causes of bias in the data-generating process
- In many cases, AI can be a powerful tool for detecting and mitigating bias, by making it visible and quantifiable in a way that human decision-making does not
- The key is to have a clear definition of fairness and a systematic process for auditing AI systems against that definition
While imperfect, the authors argue AI is often less biased than human decision-makers and offers greater potential for bias detection and mitigation. The challenge is to design systems that realize that potential.
Section: 6, Chapter: 18
Related Content
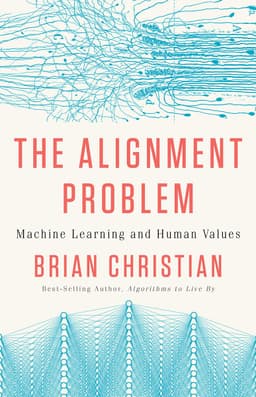
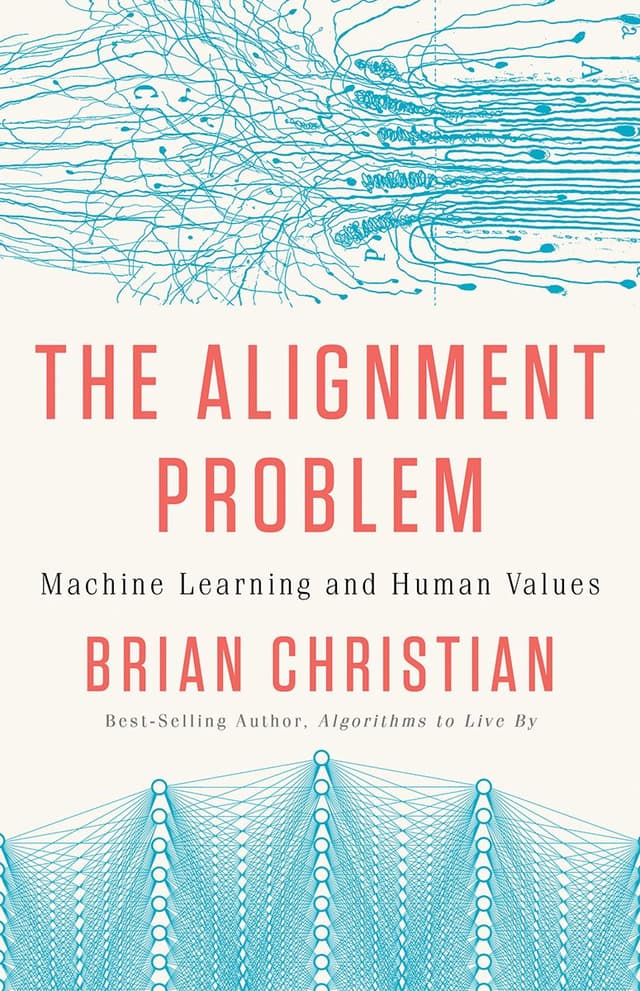
The Alignment Problem Book Summary
Brian Christian
The Alignment Problem explores the challenge of ensuring that as artificial intelligence systems grow more sophisticated, they reliably do what we want them to do - and argues that solving this "AI alignment problem" is crucial not only for beneficial AI, but for understanding intelligence and agency more broadly.
The Alignment Problem explores the challenge of ensuring that as artificial intelligence systems grow more sophisticated, they reliably do what we want them to do - and argues that solving this "AI alignment problem" is crucial not only for beneficial AI, but for understanding intelligence and agency more broadly.
Artificial Intelligence
Computer Science
Futurism