Snippets about: Prediction
Scroll left and right !
Foxy Forecasters Beat Hedgehog Historians
In his famous essay "The Hedgehog and the Fox," Isaiah Berlin argued that thinkers can be classified into two categories: Hedgehogs, who view the world through the lens of a single defining idea, and Foxes, who draw on a wide variety of experiences and perspectives.
Forecasters who were Hedgehogs - with one big theoretical view of how the world works - tended to perform quite poorly. They were overconfident and reluctant to change their minds. Foxy forecasters were much more accurate. Rather than trying to cram complex reality into a single framework, they were comfortable with cognitive dissonance and pragmatically adapted their views based on new information. Some key Fox behaviors:
- Pursuing breadth rather than depth, gathering information from diverse sources
- Aggregating many micro-theories rather than trying to build one grand theory
- Frequently using qualifying words like "however" and "on the other hand"
- Readily admitting mistakes and changing their minds
- Expressing degrees of uncertainty, rather than certainty
The Hedgehog/Fox distinction points to a crucial insight: In a complex, rapidly changing world, cognitive flexibility is more valuable than theoretical elegance. The nimble fox prevails over the stubborn hedgehog.
Section: 1, Chapter: 3
Book: Superforecasting
Author: Philip Tetlock
Beliefs Are Hypotheses To Be Tested, Not Treasures To Be Guarded
Superforecasters treat their beliefs as tentative hypotheses to be tested, rather than sacred possessions to be guarded. This is encapsulated in the idea of "actively open-minded thinking."
Some key tenets of actively open-minded thinking:
- Be willing to change your mind when presented with new evidence
- Actively seek out information that challenges your views
- Embrace uncertainty and complexity; don't be afraid to say "maybe"
- View problems from multiple perspectives; don't get wedded to one narrative
- Resist the urge to simplify and impose falsely tidy stories on reality
- Expect your beliefs to shift over time as you learn and discover your mistakes
By holding beliefs lightly, and being eager to stress-test and refine them, we can gradually move closer to the truth. Superforecasters show that this approach produces vastly better predictions compared to stubborn, overconfident ideologues.
Section: 1, Chapter: 2
Book: Superforecasting
Author: Philip Tetlock
Toward A Better Economic Forecasting Paradigm
Given the poor track record of economic forecasting to date, what would a more effective approach look like? A few key principles emerge:
- Embrace uncertainty. Rather than point forecasts, strive to quantify and communicate the full range of possible outcomes. Use probabilistic language and be explicit about your confidence level in different predictions.
- Use diverse models. Don't put all your faith in one model or method. Compare results from multiple independent approaches and be suspicious when they diverge. Use "ensemble methods" that synthesize insights from many models.
- Think in scenarios. Instead of focusing on a single "base case", map out multiple alternative futures. What has to happen for optimistic and pessimistic scenarios to play out? Which scenarios are most sensitive to your assumptions?
- Continuously update. As new data arrives, be ready to change your mind and revise your predictions. Don't get wedded to past positions. Follow the evidence where it leads, even if it's uncomfortable.
- Reward accuracy. Create incentives and accountability for forecasting precision. Keep scorecards of your prediction track record. Seek out accuracy-focused signals like prediction markets rather than just the consensus view.
Section: 1, Chapter: 3
Book: The Signal and the Noise
Author: Nate Silver
Role Models: Learning From History To Predict The Future
In the early 1970s, the U.S. government launched a mass vaccination program against the swine flu, fearing a pandemic on the scale of the 1918 Spanish flu. However, the pandemic never materialized and the vaccines caused side effects, leading to a public backlash. Silver argues this failure stemmed from health officials making predictions from limited data points without considering the full context. Role models teach the importance of learning from history's mistakes and successes to inform our predictions about the future.
Section: 1, Chapter: 7
Book: The Signal and the Noise
Author: Nate Silver
How Many Trucks Does It Take?
During World War II, the Allies wanted to estimate the number of German tanks being produced each month. Intelligence officers simply looked at serial numbers printed on captured German tanks. If tank #1,242 was built in May and tank #1,867 was built in June, then they assumed 625 tanks were built in June.
After the war, data from German factories showed that the traditional intelligence estimates were off by a factor of 2.5. But the serial number analysis was off by only 1%.
Imagine a jar filled with marbles labeled from 1 to N. You draw a random sample of marbles, noting their numbers. How can you estimate the highest number N - the total number of marbles in the jar?
Statisticians showed that the best estimator is to take the highest drawn number and multiply it by (k+1)/k, where k is the number of draws. So if you drew 4 marbles with a highest number of 78, you'd estimate there were 97.5 marbles total.
The key is that missing marbles provide information, since they indicate the jar contains numbers you haven't seen yet. The estimator performs optimally by considering both what was drawn and what likely wasn't drawn yet, given the sample. A Bayesian approach outperforms one using the observed data alone.
Section: 1, Chapter: 6
Book: Algorithms to Live By
Author: Brian Christian
The Value Of Precise Forecasts
Vague language like "a serious possibility" or "a non-negligible chance" makes it impossible to assess whether a forecast was accurate or not. In contrast, precise probabilities, like "a 62% chance", allow predictions to be unambiguously judged. Precision is necessary for forecasts to be properly tested, tracked and improved. Some key principles:
- Replace vague language with numerical odds as much as possible
- Use finely grained percentage scales (30%, 31%, 32%) rather than coarse buckets (certain, likely, toss-up, etc.)
- Specify clear time horizons and definitions for all forecast questions
- Track predictions and grade them against what actually happened
- Calculate forecasters' accuracy using quantitative measures like Brier scores
Precision takes more mental effort. But embracing it is necessary to separate lucky guesses from true skill - and to refine that skill with practice and feedback.
Section: 1, Chapter: 3
Book: Superforecasting
Author: Philip Tetlock
Belief Updating, Not IQ, Is The Core Of Superforecasting
What makes superforecasters so good? It's not their raw intelligence. The real key is how they update their beliefs in response to new information. Regular forecasters tend to be slow to change their minds, over-weighting prior views and under-weighting new data. They suffer from confirmation bias, motivated reasoning, and belief perseverance.
Superforecasters do the opposite. When new information challenges their existing views, they pounce on it and aggressively integrate it. They are always looking for reasons they could be wrong.
Belief updating is hard; it's unnatural and effortful. But superforecasters cultivate the skill through practice and repetition, like building a muscle. Over time, granular, precise updating becomes a habit.
Section: 1, Chapter: 7
Book: Superforecasting
Author: Philip Tetlock
The Hedgehog and the Fox: Two Types of Thinkers
Hedgehogs: These individuals have a single, overarching theory about the world and try to explain everything within that framework. They are confident in their predictions and resistant to changing their minds.
Foxes: These individuals are more open-minded and recognize the complexity and unpredictability of the world. They consider multiple perspectives and are more willing to adjust their views based on new information.
See Also: Range Book Summary
Section: 3, Chapter: 21
Book: Thinking, Fast and Slow
Author: Daniel Kahneman
Why We're Horrible At Detecting Terrorism
Silver argues that many of our counterterrorism efforts, like overzealous airport security, amount to "security theater" that fails to address the biggest risks. We have a bias toward stopping familiar threats, even as terrorists employ new tactics.
The TSA confiscates cigarette lighters while a terrorist could simply blow up the security line. We fear Muslims from certain countries while homegrown extremists plot undetected.
Based on a statistical analysis, Silver estimates a 3% chance of a 100,000+ fatality terror attack per decade, most likely from nuclear or biological weapons. Yet policymakers often focus more on foiling numerous small-scale conventional plots that cause less total harm.
Our brains weren't wired for such low-probability/high-impact events, so we struggle with the correct response. But we must honestly weigh the probabilities, however uncomfortable, and allocate our limited resources accordingly.
Section: 1, Chapter: 13
Book: The Signal and the Noise
Author: Nate Silver
Overcoming Our Biases With Bayesian Thinking
Silver advocates for a Bayesian approach to prediction and belief-formation. Bayes's theorem states that we should constantly update our probability estimates based on new information, weighing it against our prior assumptions. Some key takeaways:
- Explicitly quantify how probable you think something is before looking at new evidence. This prevents the common error of assigning far too much weight to a small amount of new data.
- Think probabilistically, not in binary terms. Assign levels of confidence to your beliefs rather than 100% certainty or 0% impossibility.
- Be willing to change your mind incrementally based on new information. Don't cling stubbornly to prior beliefs in the face of mounting contradictory evidence.
- Aim to steadily get closer to the truth rather than achieving perfection or claiming to have absolute knowledge. All knowledge is uncertain and subject to revision.
Section: 1, Chapter: 8
Book: The Signal and the Noise
Author: Nate Silver
Why Scouts Were Wrong About Dustin Pedroia
Red Sox star second baseman Dustin Pedroia illustrates the limits of traditional baseball scouting and the dangers of relying on conventional wisdom. Coming out of college, most scouts saw Pedroia as too small and unathletic to be a great MLB player, despite his impressive performance.
But by using comparable players and a deeper statistical analysis, forecasting systems like PECOTA saw Pedroia's true potential. Despite his unimpressive physique, Pedroia had elite bat speed, excellent plate discipline, and a stellar track record vs top competition.
Of course, the Red Sox still had to trust their own judgment enough to give Pedroia an opportunity. The point is not that data is always right and scouts are always wrong, but that forecasters need to think for themselves, dig beneath surface-level narratives, and weigh evidence in a fair-minded way.
Section: 1, Chapter: 3
Book: The Signal and the Noise
Author: Nate Silver
Successful Forecasts Are Probabilistic And Continuously Updated
Across a wide range of domains, the most accurate and useful forecasts share two key characteristics:
- They are probabilistic rather than deterministic. Instead of making a single point prediction ("GDP will grow 2.5% next year"), good forecasts provide a range and distribution of possible outcomes with associated probabilities. This honestly communicates the irreducible uncertainty around any forecast about the future. It also enables forecasters to be held accountable to results.
- Forecasts are updated continuously as new information becomes available. Static forecasts that never change are of limited use in a world where circumstances are constantly in flux. Good forecasters have the humility to change their minds in response to new facts. They understand that forecasting is an iterative process of getting closer to the truth, not an exercise in sticking to past positions.
By thinking in probabilities and continuously revising their estimates, these forecasters are able to substantially outperform "hedgehogs" who are overconfident in a single big-idea prediction.
Section: 1, Chapter: 3
Book: The Signal and the Noise
Author: Nate Silver
How Diversity Powers Prediction
A study by psychologist Jack Soll found that the average prediction of six diverse economic forecasters was 15% more accurate than even the top individual forecaster. The key was that each economist used different mental models which, when aggregated, filled in each other's blindspots. Homogenous teams of "best" forecasters who all thought alike performed worse than diverse teams with different perspectives.
Section: 1, Chapter: 2
Book: Rebel Ideas
Author: Matthew Syed
The Tip-Of-Your-Nose Perspective Is A Treacherous Guide
The "tip-of-your-nose" perspective is how we intuitively perceive the world. It refers to both
- the subjective vantage point we each have on reality, and
- the tendency to treat our personal, close-up view as the truth, even when it's distorted or missing key facts.
For example, after 9/11, many Americans felt intensely anxious about terrorism and assumed more major attacks were imminent and inevitable. The tip-of-your-nose view made it feel that way. But taking an "outside view" by comparing the 9/11 death toll to other risks like heart disease, Americans' risk of dying in a terror attack was so low it was hardly worth worrying about.
Superforecasters know the tip-of-your-nose view is frequently misleading. It may "feel right" that a company is doomed to fail or that a war is unwinnable. But feelings are not a reliable guide to reality. Only by stepping outside ourselves and stress-testing our views against data can we avoid being misled.
Section: 1, Chapter: 5
Book: Superforecasting
Author: Philip Tetlock
Mixing Human Ingenuity With Computer Power
Computers and human minds have complementary strengths in forecasting. Computers have vast data-crunching power, perform complex mathematical simulations, and tirelessly consider every possibility. However, they lack contextual understanding and can only operate based on programming from humans. Skilled human forecasters supply the intuition, hypotheses, and insights that give a model's raw output meaning and utility in the real world.
The ideal approach combines the two, using human creativity to devise strategies and programs while leveraging computational power to do the grunt work of running the numbers. An example is how weather forecasts improved dramatically once meteorologists supplemented physical models of atmospheric dynamics with computer simulations.
Section: 1, Chapter: 9
Book: The Signal and the Noise
Author: Nate Silver
What History Can (And Can't) Tell Us About The Future
Dalio cautions that even the most sophisticated forecasting has limitations. Some key principles for thinking about the future:
- It's critical to consider a wide range of possibilities and scenarios. Focus especially on the "tail risks" - low-probability, high-impact events.
- Diversification is essential. As with investing, you want a mix of bets so that you're not wiped out if any one of them goes badly wrong.
- Pay more attention to trends and trajectories than to absolute levels. The rate of change is usually more important than the current state. The US is still very powerful but its relative position is declining.
- History can be a great guide to what's possible, even if the details are impossible to predict.
Section: 3, Chapter: 14
Book: Principles For Dealing With the Changing World Order
Author: Ray Dalio
Superforecasters Aren't Afraid To Say "I Was Wrong"
One of the hardest things for any forecaster to do is to admit they were wrong. Humans are naturally resistant to acknowledging mistakes, due to cognitive dissonance and the pain of admitting error. We go to great lengths to rationalize failed predictions.
But superforecasters do the opposite. They are eager to acknowledge their misfires and examine why they happened. Some key practices:
- Meticulously tracking predictions so it's unambiguous when they fail
- Conducting "postmortems" to analyze the causes of mistakes
- Sharing lessons from failed forecasts with teammates to elevate the whole group
- Celebrating failed forecasts as learning opportunities, not shameful errors
- Revising their beliefs in light of results, even when it's uncomfortable
Superforecasters know there is no shame in being wrong. The only shame is in failing to acknowledge it or learn from it. By embracing their mistakes, they continuously sharpen their foresight.
Section: 1, Chapter: 7
Book: Superforecasting
Author: Philip Tetlock
Beware The Fallacy Of The Successful
An important lesson from Chapter 6 is to beware the fallacy of learning only from successes. This is the error of sampling on the dependent variable - looking only at the winners and trying to figure out what made them win. The problem is that this approach ignores the large number of non-winners who may have done the same things as the winners.
For example, studying only successful entrepreneurs may lead you to conclude that dropping out of college is a good idea. But this ignores the vastly larger number of college dropouts who failed to build billion-dollar businesses. The key is to study both successes and failures to identify the true factors that distinguish the two.
Section: 1, Chapter: 6
Book: The Success Equation
Author: Michael Mauboussin
Even Smart, Accomplished People Make Simple Forecasting Errors
In 1956, the respected physician Archie Cochrane was diagnosed with terminal cancer. An eminent specialist said Cochrane's axilla was "full of cancerous tissue" and he likely didn't have long to live. Cochrane immediately accepted this and started planning for death.
However, a pathologist later found no cancer in the tissue that was removed. The specialist was completely wrong. Being intelligent and accomplished was no protection against overconfidence.
Even more striking, Cochrane himself made this mistake, despite being a pioneer of evidence-based medicine. He railed against the "God complex" of physicians who relied on intuition rather than rigorous testing. Yet he blindly accepted the specialist's judgment.
Section: 1, Chapter: 2
Book: Superforecasting
Author: Philip Tetlock
The Predictability of the Future
“The idea that the future is unpredictable is undermined every day by the ease with which the past is explained.”
Section: 3, Chapter: 20
Book: Thinking, Fast and Slow
Author: Daniel Kahneman
The Signal And The Noise In Economic Data
One framework for thinking about the challenges in economic forecasting is the "signal and noise" concept. The "signal" is the true information content of economic data - the underlying trends and causal relationships we're trying to tease out. The "noise" is the random fluctuations, measurement errors, and irrelevant factors that obscure the signal.
In economic data, the noise often drowns out the signal. A few examples:
- GDP growth figures are routinely revised by multiple percentage points as new data comes in. The initial estimates are so noisy as to be nearly useless for real-time forecasting.
- Apparent patterns in things like yield curves, stock prices, or commodity prices often turn out to be random noise rather than genuine predictive signals. Statistical flukes get mistaken for meaningful economic omens.
- Economic models are built on past data that is assumed to be a fair representation of the future. But the economy's structure is constantly shifting in subtle ways. Yesterday's signal can become today's noise.
Section: 1, Chapter: 6
Book: The Signal and the Noise
Author: Nate Silver
Moneyball's Real Lesson
Many people interpreted the book and movie Moneyball to mean that statistics and quantitative analysis were a guaranteed path to success in baseball, while traditional subjective scouting was obsolete. But this is an oversimplification of the book's message.
In fact, the most successful MLB teams today employ a hybrid approach that synthesizes both scouting and statistical analysis. Even the famously data-driven Oakland A's have significantly increased their scouting budget under GM Billy Beane, recognizing the importance of data that can't be fully captured by stats.
The lesson of Moneyball is not that statistics are inherently superior to scouting or vice versa. It's that the best forecasts come from a thoughtful synthesis of both subjective and objective information. The key is having an open mind, considering multiple perspectives, and not being wedded to any one ideology. This applies far beyond baseball.
Section: 1, Chapter: 3
Book: The Signal and the Noise
Author: Nate Silver
Superforecasters Come From Diverse Backgrounds
Who are the superforecasters? They are a diverse group - engineers, lawyers, artists, scientists, Wall Streeters, and more. Many have graduate degrees, but some don't. They include a filmmaker, a mathematician, a pharmacist, and a retiree "looking to keep his mind active."
What they have in common is not so much who they are, but how they think. Superforecasters score highly on measures of fluid intelligence and actively open-minded thinking. They are numerate and capable of rapidly synthesizing information. But more important than raw intelligence is their cognitive style - they are actively open-minded, intellectually humble, eager to learn from their mistakes.
The superforecasters show that foresight isn't an innate gift, but a product of a certain way of thinking. And that way of thinking can be taught and cultivated - it doesn't require an elite background or PhD. It's an accessible skill.
Section: 1, Chapter: 4
Book: Superforecasting
Author: Philip Tetlock
Teams Of Forecasters Beat Prediction Markets
Prediction markets are often hailed as the gold standard of forecasting. These markets harness the "wisdom of crowds" by having people bet on the likelihood of future events. But teams of superforecasters did even better. Across a two year forecasting tournament, superforecaster teams bested prediction markets by 15-30%.
Why did teams do so well? The ability to share information and perspectives was key. Forecasters could share ideas, challenge each other, and collectively dig deeper into problems.
Diversity also played a big role. Teams with a variety of backgrounds and thinking styles generated more creative solutions. As long as discussions remained friendly and focused, diversity led to better accuracy.
Section: 1, Chapter: 8
Book: Superforecasting
Author: Philip Tetlock
Why We Should Express Confidence In Degrees
"When we express our beliefs (to others or just to ourselves as part of our internal decision-making dialogue), they don't generally come with qualifications. What if, in addition to expressing what we believe, we also rated our level of confidence about the accuracy of our belief on a scale of zero to ten? Zero would mean we are certain a belief is not true. Ten would mean we are certain that our belief is true. A zero-to-ten scale translates directly to percentages."
Section: 1, Chapter: 6
Book: Thinking in Bets
Author: Annie Duke
Forecasting Doesn't Require Powerful Computers Or Arcane Math
Many superforecasters have backgrounds in STEM fields and are highly numerate. They are comfortable with concepts like Bayes' theorem for updating probabilities based on new information. Some even build their own computer models.
But advanced math is not essential. Most superforecasters say they rarely use quantitative models or crunch numbers. Instead, their forecasting mainly relies on thorough research, careful reasoning, and sound judgment.
For Lionel Levine, a math professor, not using quantitative methods is a point of pride. He wants to prove he can be a great forecaster without relying on his mathematical toolkit: "It's all, you know, balancing, finding relevant information and deciding how relevant is this really? How much should it really affect my forecast?"
The key skills of balancing inside vs outside views, synthesizing perspectives, granular distinctions, and continual updating are accessible to anyone.
Section: 1, Chapter: 5
Book: Superforecasting
Author: Philip Tetlock
Beware Of Linear Models
Many economic and social science models use Gaussian/normal distributions and linear equations. However, real-world phenomena often exhibit non-linear, scalable behaviors that defy neat models.
Relying on such models can lead to massive underestimation of real risks and probabilities.
- For decisions involving uncertainty, avoid being seduced by precise equations - stay open to non-linear effects.
- Focus on the consequences of events, rather than trying to precisely model their probabilities
Section: 3, Chapter: 15
Book: The Black Swan
Author: Nassim Nicholas Taleb
Great Forecasters Have A "Scout Mindset"
The best forecasters tend to have what psychologist Julia Galef calls a "scout mindset." Think of an army scout, whose job is to accurately assess the terrain and risks ahead, in contrast to a soldier, whose job is to defeat the enemy. Forecasters with a scout mindset focus on gaining an accurate picture of reality, even when it's unpleasant or conflicts with their prior views. They are:
- Actively open-minded: Eager to test their beliefs and change their minds based on new information
- More objective: Able to separate their identity from their opinions and analyze emotionally charged issues impartially
- Comfortable with uncertainty: Accept that their knowledge is always incomplete and the future is never entirely predictable
In contrast, forecasters with a soldier mindset treat information as a weapon to defend their pre-existing beliefs. They are:
- Defensive: Emotionally attached to their opinions and quick to dismiss contrary evidence
- More biased: Allow motivated reasoning and personal agendas to skew their thinking
- Overconfident: See the future as more knowable and controllable than it is
Section: 1, Chapter: 9
Book: Superforecasting
Author: Philip Tetlock
The Catastrophic Failure Of Prediction In The 2008 Financial Crisis
he 2008 financial crisis represented a colossal failure of prediction by many of the institutions and individuals entrusted to forecast economic risk. Ratings agencies like Moody's and Standard & Poor's gave their highest AAA rating to mortgage-backed securities that were in reality extremely vulnerable to defaults. When the housing bubble burst, these securities failed at rates as high as 28%, compared to the 0.12% failure rate S&P had predicted for AAA-rated CDOs.
This predictive failure was widespread - from the ratings agencies to the banks issuing the securities to the regulators and economists who failed to sound adequate warnings. Incentive structures were poorly aligned, with entities like S&P being paid by the issuers of the securities they were rating. There was also a collective failure of imagination - an inability to consider that housing prices could decline significantly on a national basis. As a result, risks were severely underestimated, leading to the near-collapse of the global financial system when the housing bubble finally burst.
Section: 1, Chapter: 1
Book: The Signal and the Noise
Author: Nate Silver
How Weather Forecasts Have Improved Dramatically
Weather forecasting is a field where the combination of human judgment and computer modeling has led to dramatic improvements in predictive accuracy. Today, thanks to advances in computing power, data collection, and modeling techniques, weather forecasts are far more accurate than they were even 20 or 30 years ago.
For example, the average error in a hurricane forecast track has been reduced from 350 miles (for a 3-day forecast) in 1984, to just 100 miles today. Temperature and precipitation forecasts have also become much more reliable. This improved accuracy has had major benefits, giving people more time to prepare for serious storms and saving countless lives.
Weather forecasting will never be perfect due to the inherently chaotic nature of the atmosphere. But the field demonstrates that substantial progress is possible with the right combination of scientific understanding, computational firepower, and human expertise. It's a model that other disciplines can learn from.
Section: 1, Chapter: 4
Book: The Signal and the Noise
Author: Nate Silver
The Challenges Of Economic Forecasting According To Jan Hatzius
Jan Hatzius, chief economist at Goldman Sachs, encapsulates the immense difficulties inherent to economic forecasting. He cites three main challenges:
- The economy is a dynamic, constantly evolving system with complex interrelationships and feedback loops that make it very difficult to determine cause and effect from economic data alone.
- The quality of economic data is often poor, with key indicators frequently revised months or years after they are first reported. GDP growth estimates, for example, have historically been revised by an average of 1.7 percentage points.
- Because the structure of the economy is always changing, past explanations for economic behavior may not hold in the future. Economists still debate whether the Great Recession marked a fundamental "regime change" in the economy.
As a result of these challenges, even the most sophisticated economic forecasting models have poor predictive records, routinely missing major turning points in the business cycle and failing to anticipate recessions.
Section: 1, Chapter: 2
Book: The Signal and the Noise
Author: Nate Silver
The Three Measures Of A Useful Statistic
A good metric should have three key properties:
- Consistency - Does the metric reliably measure the same thing across time and contexts?
- Predictive power - Does the metric actually predict the outcome we care about?
- Noisiness - How much random variability is there in the metric relative to the signal?
The best metrics are consistent, predictive, and have a high signal-to-noise ratio. Examples of useful metrics include:
- On-base percentage in baseball (consistent, predictive of scoring, less noisy than batting average)
- Customer retention rate in business (consistent, predictive of profits, less noisy than raw sales numbers)
- Sharpe ratio in investing (consistent way to measure risk-adjusted returns, predictive of fund quality)
Section: 1, Chapter: 7
Book: The Success Equation
Author: Michael Mauboussin
Mixing Skepticism With An Open Mind
Silver argues that the best forecasters combine skepticism toward received wisdom with openness to new ideas. Some suggestions:
- Seek out thoughtful perspectives that differ from your own. Engage in good faith debates.
- Resist the urge to make snap judgments. Consider multiple hypotheses and weigh them probabilistically.
- Notice your biases and actively work to overcome them. Be intellectually humble. Use Bayesian reasoning to update your beliefs incrementally based on new information. Don't cling stubbornly to your priors.
- Focus more on honing your forecasting process than achieving specific results. Learn from mistakes and successes.
- Think in terms of nuance and degrees of uncertainty. The truth is rarely black and white.
Section: 1, Chapter: 14
Book: The Signal and the Noise
Author: Nate Silver
Three Questions To Place Any Activity On The Luck-Skill Continuum
Chapter 4 provides a simple three-question framework for placing any activity on the luck-skill continuum:
- Can you easily assign a cause to the effect you see? If yes, the activity is closer to the skill side. If not, it's closer to the luck side.
- What's the rate of reversion to the mean? The quicker the reversion, the more luck is involved.
- How predictable are the results? The more predictable, the more skill is involved.
For example, in chess, cause and effect are clear, reversion to the mean is slow, and results are highly predictable - indicating a strong role for skill. In roulette, cause and effect are unclear, reversion to the mean is rapid, and results are unpredictable - indicating a dominant role for luck. This framework provides a quick heuristic for assessing the luck-skill balance in any domain.
Section: 1, Chapter: 4
Book: The Success Equation
Author: Michael Mauboussin
"We Are All Forecasters"
"We are all forecasters. When we think about changing jobs, getting married, buying a home, making an investment, launching a product, or retiring, we decide based on how we expect the future will unfold. These expectations are forecasts."
Section: 1, Chapter: 1
Book: Superforecasting
Author: Philip Tetlock
Cultivating Supersmart Algorithms Is The Next Frontier
As powerful as superforecasters are today, the future may belong to supersmart algorithms. Human Gut may soon meet Artificial Intuition as silicon superpredictors absorb the combined wisdom of carbon-based superforecasters.
IBM's Watson, for instance, can comb through millions of medical records to predict disease progression far faster and more accurately than doctors. Similar systems could soon be forecasting currency fluctuations, climate change impacts, and election results.
Still, humans will likely remain essential - not as solo forecasters, but as partners for AI. The key will be focusing human insight on what machines can't do well: Probing assumptions, generating novel scenarios, and making meaning from raw data. The result may be an "augmented intelligence" greater than either alone.
Section: 1, Chapter: 11
Book: Superforecasting
Author: Philip Tetlock
The Perils of Market Research: Kenna's Dilemma
Gladwell introduces the story of Kenna, a talented musician whose unique sound was loved by music industry insiders but repeatedly rejected by focus groups and market research.
Kenna's music was highly praised by industry experts and fellow musicians, but his songs consistently performed poorly in market research tests. This mismatch highlights the difficulty of predicting long-term preferences from short-term exposure
Gladwell argues that snap judgments by experts can sometimes be more accurate than extensive market research. This example also challenges the common belief that more data always leads to better decisions, especially in creative fields.
Section: 1, Chapter: 5
Book: Blink
Author: Malcolm Gladwell
Grit And The Growth Mindset Are Essential For Great Forecasters
Becoming an excellent forecaster requires more than just raw intelligence. It demands the right mindset and determination. Two key traits:
- Grit - the tenacious pursuit of long-term goals in the face of adversity. Superforecasters have the dogged persistence to keep going even when the learning curve is steep and progress is slow.
- Growth Mindset - the belief that your abilities aren't fixed, but can be developed through hard work. Superforecasters view their skills not as static talents, but as muscles that grow with practice.
Both grit and a growth mindset are critical because getting great at forecasting is really hard. The world is complex and unpredictable. Feedback is slow and noisy. It can take years to measurably improve. Most people give up long before then.
But superforecasters stick with it. They have the grit to persist and the growth mindset to sustain motivation. They're energized by the challenge of getting better, one arduous step at a time. As the superforecaster Regina Schiller put it, "This is hard and I love doing it."
Section: 1, Chapter: 7
Book: Superforecasting
Author: Philip Tetlock
Small Number Of Events Explain The Majority Of Outcomes
The distribution of success isn't even - a small number of outliers have a disproportionate impact. Consider:
- Venture capital: 65% of investments lose money, 4% earn 10x+, 1% earn 50x+. That tiny minority generates most returns.
- Stock markets: Less than 10% of public companies account for all the market's gains over time.
- Art: Most works have little value, but the tiny number that are considered masterpieces drive the market.
In many fields, a tiny proportion of successes explain the vast majority of results. Success is often driven by gaining exposure to such "tail events" - outcomes that are statistically unlikely but enormously impactful.
Section: 1, Chapter: 6
Book: The Psychology of Money
Author: Morgan Housel
Superforecasters Beat The Wisdom Of The Crowd By 60%
The Good Judgment Project (GJP), led by Philip Tetlock and Barbara Mellers, recruited thousands of volunteer forecasters to predict global events as part of a tournament sponsored by the research agency IARPA. Questions covered politics, economics, national security and other topics relevant to intelligence analysts.
The GJP used multiple methods to boost forecast accuracy, including training, teaming, and statistical aggregation. But its most striking finding was that a small group of forecasters, the "superforecasters", consistently outperformed others by huge margins.
Across the first 2 years of the tournament, superforecasters beat the "wisdom of the crowd" (the average forecast of all participants) by 60% - a stunning margin. They even outperformed professional intelligence analysts with access to classified data. This suggests that generating excellent prediction accuracy doesn't require subject matter expertise or insider information - just the right cognitive skills and habits.
Section: 1, Chapter: 4
Book: Superforecasting
Author: Philip Tetlock
Taming Our Predictions
Our intuitive predictions are often overconfident and overly extreme, failing to account for the role of luck and the phenomenon of regression to the mean. Here's how to make your predictions more accurate:
Start with a Baseline Prediction: Consider the average outcome for the category or population.
Evaluate the Evidence: Assess the quality and relevance of the information you have about the specific case.
Regress Towards the Mean: Adjust your intuitive prediction towards the baseline prediction, taking into account the strength of the evidence and the degree of uncertainty.
Consider the Range of Uncertainty: Acknowledge that your prediction is not a single point but rather a range of possible outcomes.
Section: 2, Chapter: 18
Book: Thinking, Fast and Slow
Author: Daniel Kahneman
The Outside View And The Wisdom Of Crowds
The author makes the case for the "outside view" - using reference class forecasting and the wisdom of crowds to make better predictions:
- The planning fallacy: people tend to underestimate how long a project will take, going off their inside view. The outside view looks at similar projects to get a more realistic baseline.
- The optimism bias: people tend to overestimate their chances of success. The outside view looks at base rates to temper excessive optimism.
- Crowdsourcing: the average of many independent guesses is often more accurate than any individual expert's judgement. Tapping into the wisdom of crowds is a form of taking the outside view.
- Prediction markets: by aggregating many people's bets, prediction markets harness crowd wisdom to forecast everything from elections to sales figures. They beat expert forecasts across many domains.
Section: 1, Chapter: 11
Book: The Success Equation
Author: Michael Mauboussin
The Scandal of Prediction
Despite the prevalence of forecasting in domains like economics, business, and politics, the track record of "experts" in these fields is dismal. For instance, economist Edgar Fiedler found that forecasts in a sample missed the mark by an average of 2.4 times the size of changes they were trying to predict. National intelligence agencies have failed to predict nearly every major geopolitical event in the past few decades. And Philip Tetlock's seminal study on expert political forecasts found that they barely outperformed random guesses. The deeper issue is that in complex systems like economies and societies, accurate prediction is essentially impossible due to the sheer volume of interacting variables and the potential for Black Swans.
Section: 2, Chapter: 10
Book: The Black Swan
Author: Nassim Nicholas Taleb
Global Cooling And The Folly Of Prediction
In the 1970s, some scientists and media outlets warned of a looming ice age. Temperatures had been falling for decades, and some experts extrapolated this trend forward to predict cataclysmic cooling. Levitt and Dubner argue this episode carries lessons for the modern global warming debate:
- Long-term climate predictions are highly uncertain, forecasts can change rapidly as new data emerges
- Media has a tendency to hype the most alarming scenarios, glossing over uncertainty
- Myopic focus on recent data can lead to dangerous extrapolation of temporary trends
The authors note they are not suggesting global warming is another false alarm. The underlying science is far more robust today than in the 1970s. But they argue the global cooling scare demonstrates the need for epistemic humility. Levitt and Dubner believe policymakers should think probabilistically and prepare for multiple futures rather than betting everything on a single forecast.
Section: 1, Chapter: 5
Book: Super Freakonomics
Author: Steven D. Levitt , Stephen J. Dubner
Reversion To The Mean: The Cruel Equalizer
Chapter 1 also introduces the concept of reversion to the mean - the idea that an outcome that is far from the average will be followed by an outcome that is closer to the average. Reversion to the mean occurs whenever two measures are imperfectly correlated. The lower the correlation, the more extreme the reversion. In activities with a significant element of luck, reversion to the mean can be a powerful force. A few key implications:
- Don't overreact to extreme performances, good or bad. They are likely to revert toward the average.
- Be wary of paying for past performance. The "hot hand" may be nothing more than luck.
- Anticipate reversion in your own performance. If you have a great year, don't expect to maintain that level. If you have a terrible year, better times are likely ahead.
Section: 1, Chapter: 1
Book: The Success Equation
Author: Michael Mauboussin
The Simplest Models Are Often The Best
Chapter 3 makes the provocative case that often the most accurate models are the simplest ones, not complex neural networks, if the input features are wisely chosen.
Psychologist Paul Meehl showed in the 1950s that very simple statistical models consistently matched or beat expert human judgment at predicting things like academic performance or recidivism risk. Later work by Robyn Dawes in the 1970s demonstrated that even models with random feature weights (as long as they are positive) are highly competitive with human experts.
The key insight is that the predictive power comes from astute selection of the input features, not complex combinations of them. The experts' true skill is "knowing what to look for," then simple addition of those features does the rest.
This has major implications for model transparency. Wherever possible, simple, inspectable models should be preferred. And we should be extremely thoughtful about what features we choose to include since they, more than anything, drive the model's behavior.
Section: 1, Chapter: 3
Book: The Alignment Problem
Author: Brian Christian
How To Be A Little Less Certain
Manson offers some suggestions for becoming more skeptical and open-minded:
- Ask yourself "What if I'm wrong?" as often as possible. Consider alternate explanations.
- Seeing things from a different perspective takes practice. Notice when you feel threatened by new ideas.
- Argue for the other side, even if you disagree. This exercise weakens old beliefs.
- Think in terms of probabilities, not certainties. Very few things are 100% true or false.
Embracing uncertainty doesn't mean becoming totally relativistic or believing nothing. It means staying humble and curious, and being willing to update your views based on evidence. Responsible people can disagree.
Section: 1, Chapter: 6
Book: The Subtle Art of Not Giving a F*ck
Author: Mark Manson
Things That Have Never Happened Before Happen All The Time
History is a long series of events that seemed unlikely or impossible right up until they happened. This is true in investing too - the most momentous events often come out of nowhere:
- The biggest stock market crashes, like 1987 and 2008-09 had no clear warning signs
- The best investing decades often follow the worst. The 2010s bull market arose from the ashes of the financial crisis
- Innovations like the internet and smartphone have changed the world in ways few could have predicted
It's easy to use historical data to estimate the probability of future events. But never forget that the most impactful events are often ones that never happened before and seemed unimaginable at the time.
Section: 1, Chapter: 12
Book: The Psychology of Money
Author: Morgan Housel
The Land Of Heraclitean Uncertainty
In stable, slowly changing environments, past patterns can serve as reliable guides to the future. This is the "Land of Stationary Probabilities," where forecasting approaches like Nate Silver's election models work well. However, in complex, rapidly shifting contexts, the underlying rules can change abruptly, rendering historical data and models obsolete. This is the "Land of Heraclitean Uncertainty," which include such characteristics:
- Non-stationarity: The mechanisms generating outcomes vary over time.
- Non-ergodicity: Specific outcomes matter more than long-run averages.
- Non-repeatability: Situations are unique and not easily comparable to past examples.
- Contingency: Small, random factors can dramatically swing end results.
Attempting to use probabilistic methods in the Land of Heraclitean Uncertainty is akin to navigating a churning sea with a map of a still lake. Recognizing when we are in the realm of radical uncertainty rather than calculable risk is vital for effective decision-making.
Section: 1, Chapter: 6
Book: Fluke
Author: Brian Klaas
Moneyball: The Art Of Using Better Metrics
Consider the story of the Oakland A's baseball team, as chronicled in Michael Lewis's book Moneyball. For decades, baseball teams relied on traditional statistics like batting average, runs batted in, and stolen bases to evaluate players.
The A's, led by general manager Billy Beane, pioneered a new approach based on more sophisticated statistical analysis. They focused on metrics like on-base percentage and slugging percentage, which better captured a player's offensive value.
By using these better metrics, the A's were able to identify undervalued players and assemble a competitive team on a shoestring budget. Their success demonstrated the power of using the right metrics. Look for the measures that truly drive performance, even if they are unfamiliar or go against the grain.
Section: 1, Chapter: 7
Book: The Success Equation
Author: Michael Mauboussin
The Problem Of Big Data: Separating Signal From Noise
More information alone does not automatically lead to better predictions. In many fields, the growth of available data has outpaced our understanding of how to process it effectively. More data means more potential for spurious correlations, false positives, and noise obscuring the signal.
For example, the U.S. government now tracks over 45,000 economic statistics, exponentially more than even a few decades ago. But the number of genuinely causal and meaningful relationships in that data is orders of magnitude smaller. Most of it ends up being irrelevant noise when it comes to economic forecasting.
The challenge of the modern era is separating the valuable signal from the cacophony of noisy data. This requires focusing our predictions on areas where the data is most reliable and we have strong causal understanding. It also necessitates filtering the data to find the most relevant indicators.
Section: 1, Chapter: 8
Book: The Signal and the Noise
Author: Nate Silver
The Outside View Keeps Forecasters Grounded
An essential habit of superforecasters is to take the "outside view" first. This means considering a problem as an instance of a broader class, and using that class as a starting point. If you're forecasting the success of a particular startup, the outside view means first looking at the base rate of success for all startups first. If 90% of startups fail within 5 years, the outside view says there's a 90% chance this one will fail too.
Only after anchoring with the outside view do superforecasters take the "inside view" by analyzing the details of the case. If those details are exceptional, they shift the probability up or down from the base rate. But not by much - they know the outside view is usually a better guide than our internal narrative.
The outside view keeps us grounded. It prevents us from being swayed by compelling stories and overconfidently thinking "this time is different." Kahneman calls it "the single most important piece of advice regarding how to increase accuracy in forecasting."
Section: 1, Chapter: 5
Book: Superforecasting
Author: Philip Tetlock
Avoiding The Noise In Financial Markets
Financial markets produce a huge amount of noise on a day-to-day and even year-to-year basis. The price movements and endless stream of information and commentary can easily overwhelm investors' decision making. Some key lessons:
- Ignore the vast majority of short-term and medium-term price movements. Focus on the long-term underlying value of securities.
- Be wary of overtrading based on noise. Chasing short-term returns and excitement often leads to underperformance.
- The more often you check your investment returns, the more noise you expose yourself to. Have the discipline to stick to a long-term strategy.
- Diversify to reduce risk from any one investment going south. Don't put all your faith in a handful of predictions.
- Keep your emotions and biases in check. Avoid common pitfalls like overconfidence, hindsight bias, and susceptibility to stories over data.
Section: 1, Chapter: 11
Book: The Signal and the Noise
Author: Nate Silver
Conventional Beliefs Only Appear Wrong In Retrospect
What is conventionally believed and accepted as truth is very hard to see past and question when you're immersed in it. Only with hindsight do previous conventional beliefs look arbitrary and wrong. Our educational system and social status games discourage contrarian thinking. Brilliant new ideas often seem wrong or misguided at first. Having the courage to pursue them anyway, in the face of skepticism, is extremely difficult but necessary for real innovation.
Section: 1, Chapter: 2
Book: Zero to One
Author: Peter Thiel
Nobody Has A Clue
"Nobody has a clue. It's hugely difficult to forecast the business cycle. Understanding an organism as complex as the economy is very hard." - Jan Hatzius
Section: 1, Chapter: 1
Book: The Signal and the Noise
Author: Nate Silver
The Difficulty Of Predicting Earthquakes
In contrast to weather forecasting, the science of predicting earthquakes is still in its infancy. The history of the field is littered with false alarms, missed warnings, and overconfident but baseless predictions.
Seismologists have looked for predictive patterns in everything from animal behavior to electromagnetic signals to the timing of foreshocks. But none of these methods have delivered reliable predictions. The fundamental problem is that earthquake dynamics are extremely complex, nonlinear, and difficult to model. Current quake models are like "black boxes" - they can fit past data but have little predictive power.
Some argue that earthquakes are inherently unpredictable - a chaotic system where small changes in initial conditions can lead to vastly different outcomes. The jury is still out, but the track record to date suggests that reliable earthquake prediction is an extremely difficult challenge that will not be solved anytime soon.
Section: 1, Chapter: 5
Book: The Signal and the Noise
Author: Nate Silver
Predicting the Future
“Predicting what the world will look like fifty years from now is impossible. But predicting that people will still respond to greed, fear, opportunity, exploitation, risk, uncertainty, tribal affiliations, and social persuasion in the same way is a bet I’d take.”
Section: 1, Chapter: 2
Book: Same as Ever
Author: Morgan Housel
Why It's Hard To Assess Forecast Accuracy
Steve Ballmer's infamous 2007 forecast that "There's no chance that the iPhone is going to get any significant market share" looks hugely wrong in hindsight. But Ballmer never specified what "significant" market share meant, or what time period he was referring to. His forecast was too vague to definitely judge as right or wrong.
This is extremely common - and makes it effectively impossible to assess forecast accuracy. To be testable, forecasts need:
- Specific definitions. What counts as a "default" or a "bubble" or a "coup"?
- Precise time horizons. By what date will the event happen or not?
- Numerical probabilities that can be scored. "60% chance" can be graded later as right or wrong; "pretty likely" cannot.
- Repeated forecasts over time. One forecast is not enough - we need a track record.
Most real-world forecasts fail these criteria. As a result, we have little idea how accurate experts actually are, despite how much influence their predictions have.
Section: 1, Chapter: 3
Book: Superforecasting
Author: Philip Tetlock
Humans + Computers + Common Sense
The future of forecasting across many domains will be a synthesis of human judgment, computer power and models, and plain old common sense and historical perspective. Expert forecasters like Hatzius and Hough don't just rely on equations - they think deeply about the data, put it in appropriate context, and weigh risks in a balanced way. While Big Data and AI will continue to advance, there is still no substitute for human wisdom and experience in navigating the signal and the noise.
Section: 1, Chapter: 3
Book: The Signal and the Noise
Author: Nate Silver
"The Problem Of False Positives"
"But the number of meaningful relationships in the data—those that speak to causality rather than correlation and testify to how the world really works—is orders of magnitude smaller. Nor is it likely to be increasing at nearly so fast a rate as the information itself; there isn't any more truth in the world than there was before the Internet or the printing press. Most of the data is just noise, as most of the universe is filled with empty space."
Section: 1, Chapter: 8
Book: The Signal and the Noise
Author: Nate Silver
Beware Overconfident Forecasts - Economists' Poor Track Record Of Predicting Recessions
One of the clearest lessons from economic history is to be deeply skeptical of overconfident economic forecasts, especially those that proclaim a "new era" of uninterrupted growth or that project present trends indefinitely into the future. Economists have a dismal record of predicting recessions and major turning points in the business cycle.
In the 2007-2008 financial crisis, for example, the median forecast from leading economists was that the economy would avoid recession and continue to grow. Even once the recession had already begun in December 2007, most economists still thought a recession was unlikely.
Part of the problem is incentives - bearish forecasts are often punished by markets and by clients who don't want to believe the party will ever end. There are also psychological biases at play, like recency bias (putting too much weight on recent events and performance) and overconfidence. Any projection that doesn't grapple with uncertainty and discuss the many ways the forecaster could be wrong is not worth very much.
Section: 1, Chapter: 2
Book: The Signal and the Noise
Author: Nate Silver
WYSIATI Explains Why We Jump To Conclusions
WYSIATI (What You See Is All There Is) is a key mental trap that leads to flawed predictions. It refers to our mind's tendency to draw firm conclusions from whatever limited information is available, rather than recognizing the information we don't have.
For example, after the 2011 Norway terrorist attacks, many people immediately assumed Islamist terrorists were responsible, based on recent events like 9/11 and the bits of evidence available, like the scale of the attacks. However, the perpetrator turned out to be a right-wing anti-Muslim extremist, Anders Breivik.
WYSIATI explains why we jump to conclusions rather than saying "I don't know" or "I need more information." Our minds abhor uncertainty. We impose coherent narratives on events, even when key facts are missing. Breaking this habit is crucial to forecasting better.
Section: 1, Chapter: 2
Book: Superforecasting
Author: Philip Tetlock
"Diversity Matters As Much As Ability"
"What matters is having people who think differently and have different points of information, and this is really important. Having a group of really smart people who tend to see the world the same way and process information the same way isn't nearly as effective as a more diverse team." - Jonathan Baron
Section: 1, Chapter: 6
Book: Superforecasting
Author: Philip Tetlock
"It was so unexpected"
"It was so unexpected," we will say. We will spend considerable energy convincing ourselves that it was so unexpected, not foreseeable, and unpredictable. Unpredicted? Yes. Unpredictable? Not necessarily... Our track record in predicting those events is dismal; yet by some mechanism called the hindsight bias we think that we understand them."
Section: 1, Chapter: 1
Book: The Black Swan
Author: Nassim Nicholas Taleb
How To Think About Reversion To The Mean
Mauboussin offers practical advice on how to use the concept of reversion to the mean to make better predictions and decisions:
- Reversion to the mean is a statistical reality in any system where two measures are imperfectly correlated. Extreme outcomes tend to be followed by more average outcomes.
- The key to using reversion to the mean is to know where the mean is. In other words, you need a sense of the underlying base rate or long-term average. Err on the side of the mean.
- The more extreme the initial outcome and the further it is from the mean, the more you should expect it to revert.
Section: 1, Chapter: 10
Book: The Success Equation
Author: Michael Mauboussin
Break Big Problems Down Using Fermi Estimates
To make impossibly complex problems tractable, superforecasters often use "Fermi-style" analysis, named after the physicist Enrico Fermi. The steps:
- Clearly specify the thing you want to predict (e.g. "How many piano tuners are there in Chicago?")
- Break the problem down into smaller, easier parts. ("How many pianos are there in Chicago? How often are they tuned each year? How many can one tuner service per year?")
- Make a reasonable guess for each component, based on whatever information you have or can gather. Focus on quantities you can approximate, even if crudely.
- Combine your component estimates into an overall estimate, using simple math (e.g. # of pianos * # of tunings per piano per year / # of tunings per tuner per year = # of tuners)
The resulting estimate won't be exact, but it's often surprisingly close - and much better than a wild guess. By breaking big mysteries down into small, knowable parts, Fermi estimates make unknowns more manageable.
Section: 1, Chapter: 5
Book: Superforecasting
Author: Philip Tetlock
The Skeptic And The Optimist
Philip Tetlock considers himself an "optimistic skeptic" when it comes to forecasting. The skeptical side recognizes the huge challenges of predicting the future in a complex, nonlinear world. Even small unpredictable events, like the self-immolation of a Tunisian fruit vendor, can have cascading consequences no one foresaw, like the Arab Spring uprisings.
However, the optimistic side believes foresight is possible, to some degree, in some circumstances. We make mundane forecasts constantly in everyday life. Sophisticated forecasts underpin things like insurance and inventory management. The key is to figure out what makes forecasts more or less accurate, by gathering many forecasts, measuring accuracy, and rigorously analyzing results. This is rarely done today - but it can be.
Section: 1, Chapter: 1
Book: Superforecasting
Author: Philip Tetlock
Books about Prediction
Prediction
Decision Making
Thinking
Leadership
Superforecasting Book Summary
Philip Tetlock
In Superforecasting, Philip Tetlock and Dan Gardner reveal the techniques used by elite forecasters to predict future events with remarkable accuracy, and show how these skills can be cultivated by anyone to make better decisions in an uncertain world.
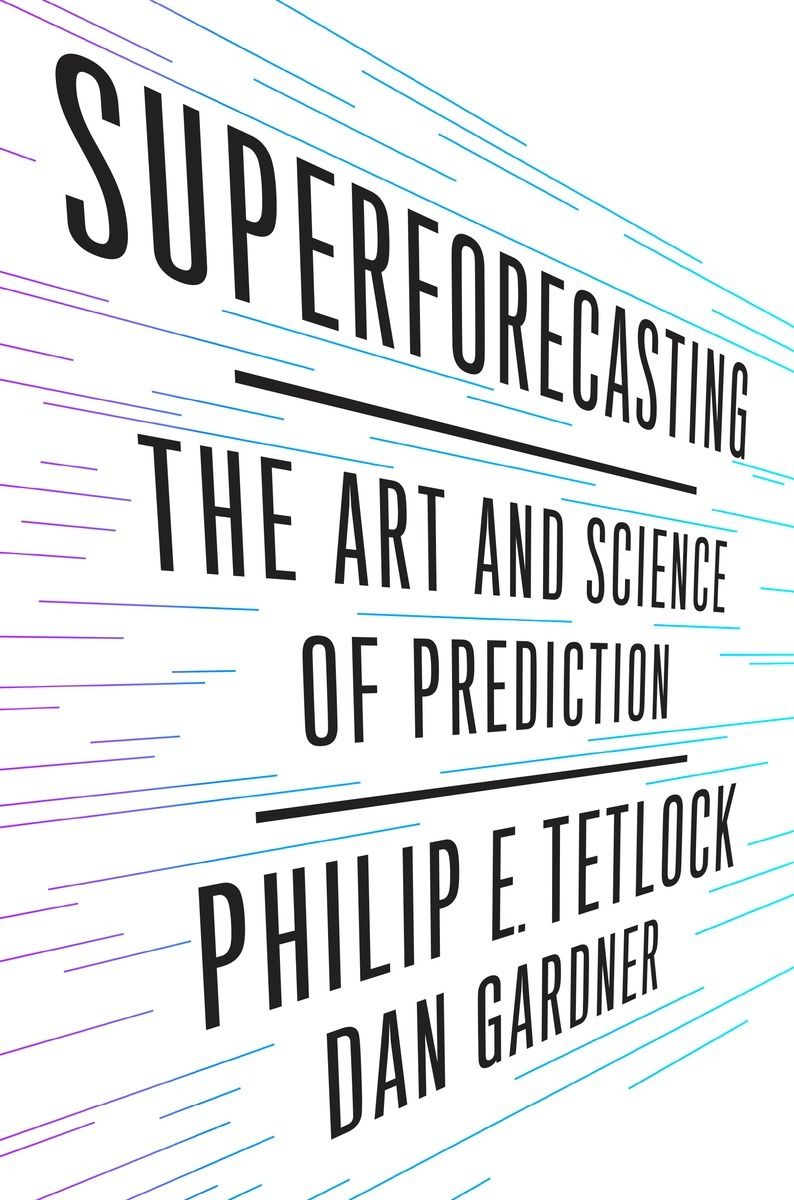
The Black Swan Book Summary
Nassim Nicholas Taleb
The Black Swan is about the extreme impact of rare and unpredictable outlier events, and how we tend to find simplistic explanations for them after the fact, making us blind to randomness and vulnerable to future Black Swans.
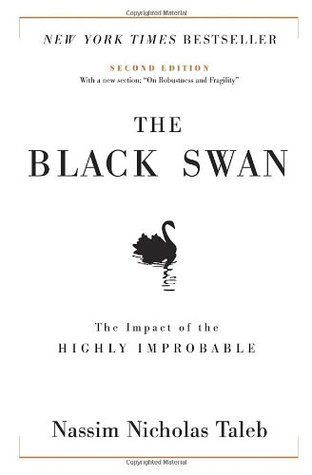
Prediction
Decision Making
Economics
The Signal and the Noise Book Summary
Nate Silver
In The Signal and the Noise, Nate Silver explores the art and science of prediction, explaining what separates good forecasters from bad ones and how we can all improve our understanding of an uncertain world.
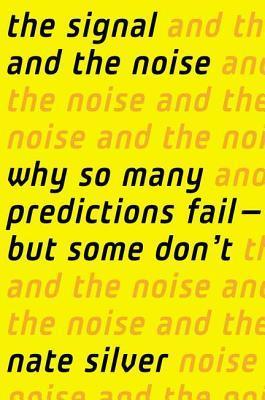
Economics
Decision Making
Prediction
Game Theory
On The Edge Book Summary
Nate Silver
"On the Edge" takes readers on a captivating journey through the high-stakes world of poker, sports betting, and risk-taking, revealing the counterintuitive strategies and mental habits that allow gamblers and daredevils to thrive in the face of daunting odds.
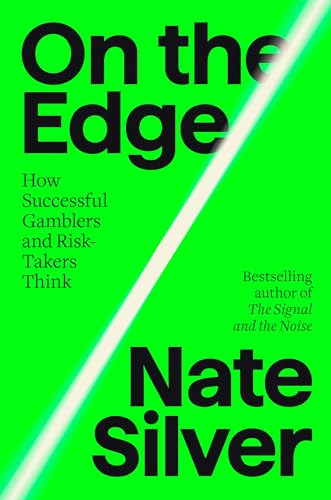