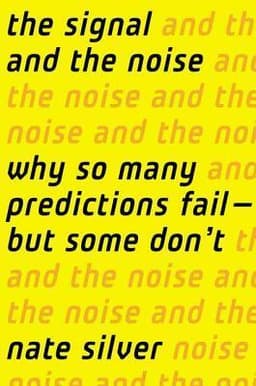
The Signal and the Noise Book Summary
Why So Many Predictions Fail—But Some Don't
Book by Nate Silver
Summary
In The Signal and the Noise, Nate Silver explores the art and science of prediction, explaining what separates good forecasters from bad ones and how we can all improve our understanding of an uncertain world.
Sign in to rate
Average Rating: 5
The Catastrophic Failure Of Prediction In The 2008 Financial Crisis
he 2008 financial crisis represented a colossal failure of prediction by many of the institutions and individuals entrusted to forecast economic risk. Ratings agencies like Moody's and Standard & Poor's gave their highest AAA rating to mortgage-backed securities that were in reality extremely vulnerable to defaults. When the housing bubble burst, these securities failed at rates as high as 28%, compared to the 0.12% failure rate S&P had predicted for AAA-rated CDOs.
This predictive failure was widespread - from the ratings agencies to the banks issuing the securities to the regulators and economists who failed to sound adequate warnings. Incentive structures were poorly aligned, with entities like S&P being paid by the issuers of the securities they were rating. There was also a collective failure of imagination - an inability to consider that housing prices could decline significantly on a national basis. As a result, risks were severely underestimated, leading to the near-collapse of the global financial system when the housing bubble finally burst.
Section: 1, Chapter: 1
Nobody Has A Clue
"Nobody has a clue. It's hugely difficult to forecast the business cycle. Understanding an organism as complex as the economy is very hard." - Jan Hatzius
Section: 1, Chapter: 1
The Productivity Paradox And The Lag Between Technology And Productivity
The history of technology adoption is full of examples where the expected productivity benefits of a major innovation were slow to materialize - a phenomenon termed the "productivity paradox." In the 1970s and 1980s, for example, businesses made huge investments in computers and information technology. But productivity growth actually slowed during this period, puzzling economists.
It took a long time for businesses to figure out how to reorganize their processes and train their workforces to take full advantage of computers.
We're seeing a version of this story play out with Big Data and AI today. Despite the hype about these technologies revolutionizing every industry, hard productivity numbers have yet to catch up with the promised potential. That doesn't mean the revolution won't happen - just that it will likely take longer than expected as businesses gradually learn how to fully harness the power of these innovations.
Section: 1, Chapter: 1
The Challenges Of Economic Forecasting According To Jan Hatzius
Jan Hatzius, chief economist at Goldman Sachs, encapsulates the immense difficulties inherent to economic forecasting. He cites three main challenges:
- The economy is a dynamic, constantly evolving system with complex interrelationships and feedback loops that make it very difficult to determine cause and effect from economic data alone.
- The quality of economic data is often poor, with key indicators frequently revised months or years after they are first reported. GDP growth estimates, for example, have historically been revised by an average of 1.7 percentage points.
- Because the structure of the economy is always changing, past explanations for economic behavior may not hold in the future. Economists still debate whether the Great Recession marked a fundamental "regime change" in the economy.
As a result of these challenges, even the most sophisticated economic forecasting models have poor predictive records, routinely missing major turning points in the business cycle and failing to anticipate recessions.
Section: 1, Chapter: 2
Beware Overconfident Forecasts - Economists' Poor Track Record Of Predicting Recessions
One of the clearest lessons from economic history is to be deeply skeptical of overconfident economic forecasts, especially those that proclaim a "new era" of uninterrupted growth or that project present trends indefinitely into the future. Economists have a dismal record of predicting recessions and major turning points in the business cycle.
In the 2007-2008 financial crisis, for example, the median forecast from leading economists was that the economy would avoid recession and continue to grow. Even once the recession had already begun in December 2007, most economists still thought a recession was unlikely.
Part of the problem is incentives - bearish forecasts are often punished by markets and by clients who don't want to believe the party will ever end. There are also psychological biases at play, like recency bias (putting too much weight on recent events and performance) and overconfidence. Any projection that doesn't grapple with uncertainty and discuss the many ways the forecaster could be wrong is not worth very much.
Section: 1, Chapter: 2
Moneyball's Real Lesson
Many people interpreted the book and movie Moneyball to mean that statistics and quantitative analysis were a guaranteed path to success in baseball, while traditional subjective scouting was obsolete. But this is an oversimplification of the book's message.
In fact, the most successful MLB teams today employ a hybrid approach that synthesizes both scouting and statistical analysis. Even the famously data-driven Oakland A's have significantly increased their scouting budget under GM Billy Beane, recognizing the importance of data that can't be fully captured by stats.
The lesson of Moneyball is not that statistics are inherently superior to scouting or vice versa. It's that the best forecasts come from a thoughtful synthesis of both subjective and objective information. The key is having an open mind, considering multiple perspectives, and not being wedded to any one ideology. This applies far beyond baseball.
Section: 1, Chapter: 3
Why Scouts Were Wrong About Dustin Pedroia
Red Sox star second baseman Dustin Pedroia illustrates the limits of traditional baseball scouting and the dangers of relying on conventional wisdom. Coming out of college, most scouts saw Pedroia as too small and unathletic to be a great MLB player, despite his impressive performance.
But by using comparable players and a deeper statistical analysis, forecasting systems like PECOTA saw Pedroia's true potential. Despite his unimpressive physique, Pedroia had elite bat speed, excellent plate discipline, and a stellar track record vs top competition.
Of course, the Red Sox still had to trust their own judgment enough to give Pedroia an opportunity. The point is not that data is always right and scouts are always wrong, but that forecasters need to think for themselves, dig beneath surface-level narratives, and weigh evidence in a fair-minded way.
Section: 1, Chapter: 3
Successful Forecasts Are Probabilistic And Continuously Updated
Across a wide range of domains, the most accurate and useful forecasts share two key characteristics:
- They are probabilistic rather than deterministic. Instead of making a single point prediction ("GDP will grow 2.5% next year"), good forecasts provide a range and distribution of possible outcomes with associated probabilities. This honestly communicates the irreducible uncertainty around any forecast about the future. It also enables forecasters to be held accountable to results.
- Forecasts are updated continuously as new information becomes available. Static forecasts that never change are of limited use in a world where circumstances are constantly in flux. Good forecasters have the humility to change their minds in response to new facts. They understand that forecasting is an iterative process of getting closer to the truth, not an exercise in sticking to past positions.
By thinking in probabilities and continuously revising their estimates, these forecasters are able to substantially outperform "hedgehogs" who are overconfident in a single big-idea prediction.
Section: 1, Chapter: 3
This concept is also discussed in:
Range
Humans + Computers + Common Sense
The future of forecasting across many domains will be a synthesis of human judgment, computer power and models, and plain old common sense and historical perspective. Expert forecasters like Hatzius and Hough don't just rely on equations - they think deeply about the data, put it in appropriate context, and weigh risks in a balanced way. While Big Data and AI will continue to advance, there is still no substitute for human wisdom and experience in navigating the signal and the noise.
Section: 1, Chapter: 3
How Weather Forecasts Have Improved Dramatically
Weather forecasting is a field where the combination of human judgment and computer modeling has led to dramatic improvements in predictive accuracy. Today, thanks to advances in computing power, data collection, and modeling techniques, weather forecasts are far more accurate than they were even 20 or 30 years ago.
For example, the average error in a hurricane forecast track has been reduced from 350 miles (for a 3-day forecast) in 1984, to just 100 miles today. Temperature and precipitation forecasts have also become much more reliable. This improved accuracy has had major benefits, giving people more time to prepare for serious storms and saving countless lives.
Weather forecasting will never be perfect due to the inherently chaotic nature of the atmosphere. But the field demonstrates that substantial progress is possible with the right combination of scientific understanding, computational firepower, and human expertise. It's a model that other disciplines can learn from.
Section: 1, Chapter: 4
The Difficulty Of Predicting Earthquakes
In contrast to weather forecasting, the science of predicting earthquakes is still in its infancy. The history of the field is littered with false alarms, missed warnings, and overconfident but baseless predictions.
Seismologists have looked for predictive patterns in everything from animal behavior to electromagnetic signals to the timing of foreshocks. But none of these methods have delivered reliable predictions. The fundamental problem is that earthquake dynamics are extremely complex, nonlinear, and difficult to model. Current quake models are like "black boxes" - they can fit past data but have little predictive power.
Some argue that earthquakes are inherently unpredictable - a chaotic system where small changes in initial conditions can lead to vastly different outcomes. The jury is still out, but the track record to date suggests that reliable earthquake prediction is an extremely difficult challenge that will not be solved anytime soon.
Section: 1, Chapter: 5
The Signal And The Noise In Economic Data
One framework for thinking about the challenges in economic forecasting is the "signal and noise" concept. The "signal" is the true information content of economic data - the underlying trends and causal relationships we're trying to tease out. The "noise" is the random fluctuations, measurement errors, and irrelevant factors that obscure the signal.
In economic data, the noise often drowns out the signal. A few examples:
- GDP growth figures are routinely revised by multiple percentage points as new data comes in. The initial estimates are so noisy as to be nearly useless for real-time forecasting.
- Apparent patterns in things like yield curves, stock prices, or commodity prices often turn out to be random noise rather than genuine predictive signals. Statistical flukes get mistaken for meaningful economic omens.
- Economic models are built on past data that is assumed to be a fair representation of the future. But the economy's structure is constantly shifting in subtle ways. Yesterday's signal can become today's noise.
Section: 1, Chapter: 6
Bias And Incentive Problems In Economic Forecasting
Economic forecasts also suffer from bias and misaligned incentives. Even when forecasters have access to the same data, their predictions are often skewed by unconscious biases and career pressures.
One common bias is overconfidence. Forecasters (and their clients) often put too much faith in complex econometric models, ignoring the uncertainties in their assumptions and the economy's inherent unpredictability. Many forecasts are presented as precise point estimates rather than ranges, creating a false sense of certainty.
Incentives also play a role. In a famous experiment, Harvard undergrads were divided into "buyers" and "sellers" of a hypothetical asset. The catch was that sellers were given a token reward if the asset price stayed high. This small incentive was enough to make sellers significantly more bullish in their price predictions vs buyers. The same dynamic plays out in the real economy - analysts affiliated with financial firms tend to make rosier GDP forecasts than independent academics.
Section: 1, Chapter: 6
Toward A Better Economic Forecasting Paradigm
Given the poor track record of economic forecasting to date, what would a more effective approach look like? A few key principles emerge:
- Embrace uncertainty. Rather than point forecasts, strive to quantify and communicate the full range of possible outcomes. Use probabilistic language and be explicit about your confidence level in different predictions.
- Use diverse models. Don't put all your faith in one model or method. Compare results from multiple independent approaches and be suspicious when they diverge. Use "ensemble methods" that synthesize insights from many models.
- Think in scenarios. Instead of focusing on a single "base case", map out multiple alternative futures. What has to happen for optimistic and pessimistic scenarios to play out? Which scenarios are most sensitive to your assumptions?
- Continuously update. As new data arrives, be ready to change your mind and revise your predictions. Don't get wedded to past positions. Follow the evidence where it leads, even if it's uncomfortable.
- Reward accuracy. Create incentives and accountability for forecasting precision. Keep scorecards of your prediction track record. Seek out accuracy-focused signals like prediction markets rather than just the consensus view.
Section: 1, Chapter: 3
Role Models: Learning From History To Predict The Future
In the early 1970s, the U.S. government launched a mass vaccination program against the swine flu, fearing a pandemic on the scale of the 1918 Spanish flu. However, the pandemic never materialized and the vaccines caused side effects, leading to a public backlash. Silver argues this failure stemmed from health officials making predictions from limited data points without considering the full context. Role models teach the importance of learning from history's mistakes and successes to inform our predictions about the future.
Section: 1, Chapter: 7
"The Problem Of False Positives"
"But the number of meaningful relationships in the data—those that speak to causality rather than correlation and testify to how the world really works—is orders of magnitude smaller. Nor is it likely to be increasing at nearly so fast a rate as the information itself; there isn't any more truth in the world than there was before the Internet or the printing press. Most of the data is just noise, as most of the universe is filled with empty space."
Section: 1, Chapter: 8
Overcoming Our Biases With Bayesian Thinking
Silver advocates for a Bayesian approach to prediction and belief-formation. Bayes's theorem states that we should constantly update our probability estimates based on new information, weighing it against our prior assumptions. Some key takeaways:
- Explicitly quantify how probable you think something is before looking at new evidence. This prevents the common error of assigning far too much weight to a small amount of new data.
- Think probabilistically, not in binary terms. Assign levels of confidence to your beliefs rather than 100% certainty or 0% impossibility.
- Be willing to change your mind incrementally based on new information. Don't cling stubbornly to prior beliefs in the face of mounting contradictory evidence.
- Aim to steadily get closer to the truth rather than achieving perfection or claiming to have absolute knowledge. All knowledge is uncertain and subject to revision.
Section: 1, Chapter: 8
The Problem Of Big Data: Separating Signal From Noise
More information alone does not automatically lead to better predictions. In many fields, the growth of available data has outpaced our understanding of how to process it effectively. More data means more potential for spurious correlations, false positives, and noise obscuring the signal.
For example, the U.S. government now tracks over 45,000 economic statistics, exponentially more than even a few decades ago. But the number of genuinely causal and meaningful relationships in that data is orders of magnitude smaller. Most of it ends up being irrelevant noise when it comes to economic forecasting.
The challenge of the modern era is separating the valuable signal from the cacophony of noisy data. This requires focusing our predictions on areas where the data is most reliable and we have strong causal understanding. It also necessitates filtering the data to find the most relevant indicators.
Section: 1, Chapter: 8
Mixing Human Ingenuity With Computer Power
Computers and human minds have complementary strengths in forecasting. Computers have vast data-crunching power, perform complex mathematical simulations, and tirelessly consider every possibility. However, they lack contextual understanding and can only operate based on programming from humans. Skilled human forecasters supply the intuition, hypotheses, and insights that give a model's raw output meaning and utility in the real world.
The ideal approach combines the two, using human creativity to devise strategies and programs while leveraging computational power to do the grunt work of running the numbers. An example is how weather forecasts improved dramatically once meteorologists supplemented physical models of atmospheric dynamics with computer simulations.
Section: 1, Chapter: 9
The Poker Bubble: Lessons From The Boom And Bust
In the early 2000s, online poker sites experienced a massive boom in popularity and profitability. Chris Moneymaker's surprise win at the 2003 World Series of Poker helped drive a surge of new amateur players to online poker.
However, the U.S. government cracked down on online poker in 2006, causing a sharp decline in casual players. As the "fish" disappeared, the games got tougher and more professionals struggled to make a consistent profit. Many sites eventually became insolvent or shut down.
Silver draws parallels to financial market bubbles and how the poker ecosystem became unstable as the loose money dried up. The poker boom and bust cycle illustrates how predictions can go awry when market conditions change and uncertainty increases.
Section: 1, Chapter: 10
Avoiding The Noise In Financial Markets
Financial markets produce a huge amount of noise on a day-to-day and even year-to-year basis. The price movements and endless stream of information and commentary can easily overwhelm investors' decision making. Some key lessons:
- Ignore the vast majority of short-term and medium-term price movements. Focus on the long-term underlying value of securities.
- Be wary of overtrading based on noise. Chasing short-term returns and excitement often leads to underperformance.
- The more often you check your investment returns, the more noise you expose yourself to. Have the discipline to stick to a long-term strategy.
- Diversify to reduce risk from any one investment going south. Don't put all your faith in a handful of predictions.
- Keep your emotions and biases in check. Avoid common pitfalls like overconfidence, hindsight bias, and susceptibility to stories over data.
Section: 1, Chapter: 11
Why We're Horrible At Detecting Terrorism
Silver argues that many of our counterterrorism efforts, like overzealous airport security, amount to "security theater" that fails to address the biggest risks. We have a bias toward stopping familiar threats, even as terrorists employ new tactics.
The TSA confiscates cigarette lighters while a terrorist could simply blow up the security line. We fear Muslims from certain countries while homegrown extremists plot undetected.
Based on a statistical analysis, Silver estimates a 3% chance of a 100,000+ fatality terror attack per decade, most likely from nuclear or biological weapons. Yet policymakers often focus more on foiling numerous small-scale conventional plots that cause less total harm.
Our brains weren't wired for such low-probability/high-impact events, so we struggle with the correct response. But we must honestly weigh the probabilities, however uncomfortable, and allocate our limited resources accordingly.
Section: 1, Chapter: 13
Mixing Skepticism With An Open Mind
Silver argues that the best forecasters combine skepticism toward received wisdom with openness to new ideas. Some suggestions:
- Seek out thoughtful perspectives that differ from your own. Engage in good faith debates.
- Resist the urge to make snap judgments. Consider multiple hypotheses and weigh them probabilistically.
- Notice your biases and actively work to overcome them. Be intellectually humble. Use Bayesian reasoning to update your beliefs incrementally based on new information. Don't cling stubbornly to your priors.
- Focus more on honing your forecasting process than achieving specific results. Learn from mistakes and successes.
- Think in terms of nuance and degrees of uncertainty. The truth is rarely black and white.
Section: 1, Chapter: 14
Related Content
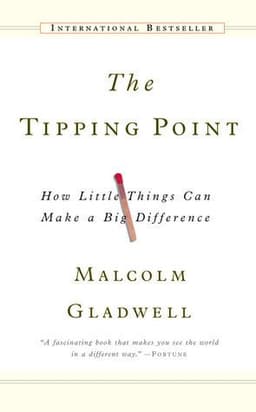
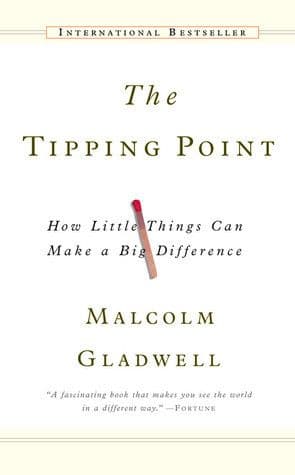
The Tipping Point Book Summary
Malcolm Gladwell
The Tipping Point reveals the hidden forces that cause social epidemics - from fashion trends to crime waves - to spread like viruses, and shows how to start and control positive epidemics of our own.
The Tipping Point reveals the hidden forces that cause social epidemics - from fashion trends to crime waves - to spread like viruses, and shows how to start and control positive epidemics of our own.
Psychology
Personal Development
Sociology
Economics
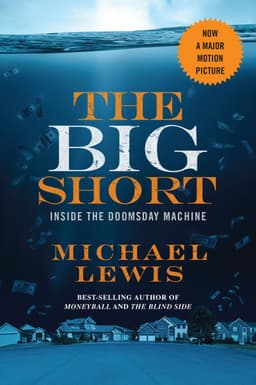
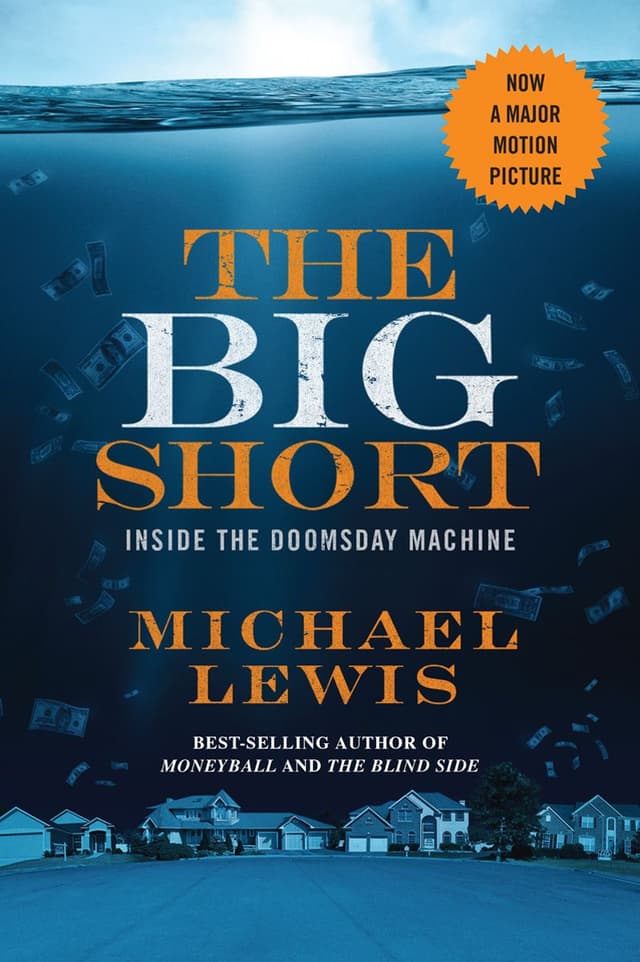
The Big Short Book Summary
Michael Lewis
In "The Big Short," Michael Lewis tells the story of the contrarian investors who predicted the subprime mortgage crisis and made a fortune betting against the bubble, exposing the greed, delusion, and perverse incentives that nearly brought down the global financial system.
In "The Big Short," Michael Lewis tells the story of the contrarian investors who predicted the subprime mortgage crisis and made a fortune betting against the bubble, exposing the greed, delusion, and perverse incentives that nearly brought down the global financial system.
Finance
Economics
Money
Biography